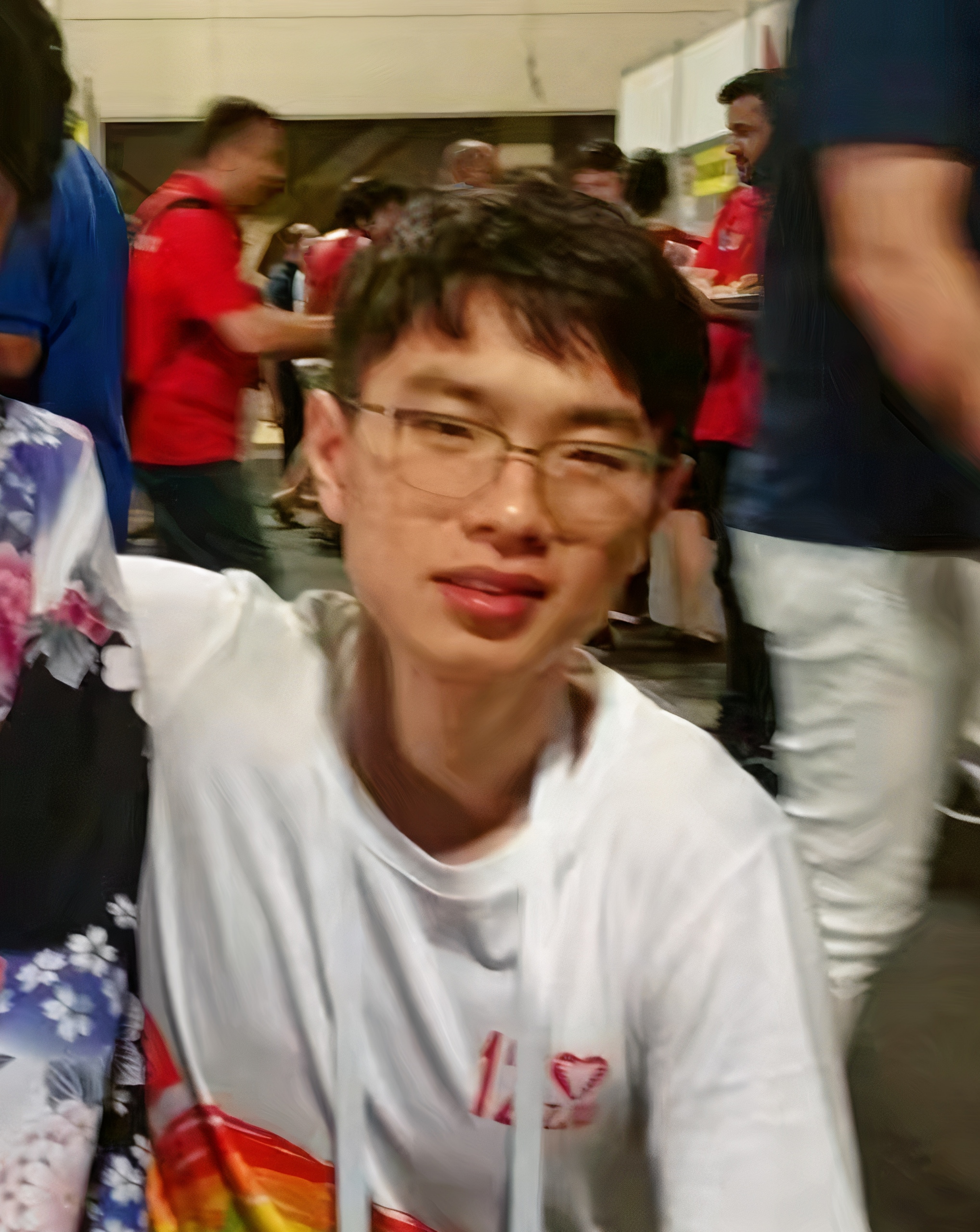
Huaiyang HUANG
I am a Ph.D. candidate in the Department of Computer Science and Engineering, of RAM-Lab at Robotics Institute, the Hong Kong
University of Science and Technology,
supervised by Prof. Ming LIU. I received my B.E. degree in Mechatronics
from Zhejiang University, where I was a member of robot
soccer team ZJUDancer.
In the past years, I mainly work on localization,
mapping and 3D
reconstruction with various sensor configurations. In particular, I am interested in cutting-edge
technologies for geometry-related problems in autonomous navigation and 3D vision.
Reasearch Projects
Robust Localization Aided by Dense Scene Geometry
Developing a system that localizes and tracks a low-cost sensor suite (e.g., monocular-inertial sensor) within a accurate dense map building by laser scanners. To alleviate challenges (illumination change, view-point variances, etc) in feature-based visual localization pipeline, this project introduces dense scene geometry in the process of visual localization. Various dense map representation are investigated for localization, including Signed Distance Field (SDF), Surfel Map and Gaussian Mixture Model (GMM). In addition to dense structure, other modalities like inertial measurements can also be fused into the localization process. For details please see the links below.
Related Publications:
Metric Monocular Localization Using Signed Distance
Fields.
Huaiyang Huang, Yuxiang Sun, Haoyang Ye, Ming Liu.
IEEE/RSJ International Conference on Intelligent Robots and Systems (IROS)
, 2019.
Monocular Direct Sparse Localization
in a Prior 3D Surfel
Map.
Huaiyang Huang, Yuxiang Sun, Haoyang Ye, Ming Liu.
IEEE International Conference on Robotics and Automation (ICRA)
, 2020.
GMMLoc: Structure Consistent Visual Localization with
Gaussian Mixture Model.
Huaiyang Huang,Haoyang Ye, Yuxiang Sun, Ming Liu.
IEEE Robotics and Automation Letters (RA-L)
, 2020.
Localization and Mapping with Diverse Sensor Configuration
Developing localization and mapping systems with diverse sensor configurations, including cameras, LiDAR, IMU, wheeled odometer etc. Firstly, we develop a system that efficiently builds a dense map with multiple point cloud scans. Instead of using traditional scan-to-model or pose synchronization-based pipeline, the system introduces a novel formulation of point cloud Bundle Adjustment (BA), which improves the local consistency of map while maintaining better efficiency. The system is then extended to a LiDAR-based SLAM system with a visibility graph between map component and frames and a deformation-based loop correction module. Global map consistency cloud be well maintained in a campus-level dataset by the proposed system. The system achieves SOTA performance on public dataset and ranks 8-th on KITTI Odometry benchmark. In addition, we introduce learnt features into visual SLAM systems. We propose a two-stage direct tracking method by maximizing the predicted repeatability of landmarks. The system achieves sota performance in public datasets KITTI Odometry and EuRoC. We also extend the system into a localization system and performed several tests in various real-world scenarios, including public road, campus and harbor. For details please see links below.
Related Publications:
On Bundle Adjustment for Multiview Point Cloud
Registration.
Huaiyang Huang, Yuxiang Sun, Jin Wu, Jianhao Jiao, Xiangcheng Hu, Linwei Zheng, Lujia Wang, Ming Liu.
IEEE Robotics and Automation Letters (RA-L)
, 2021.
Incorporating Learnt Local and Global Embeddings into
Monocular Visual SLAM
Huaiyang Huang, Haoyang Ye, Yuxiang Sun, Ming Liu.
Autonomous Robots (AURO)
, 2020.
Monocular Visual Odometry using Learned Repeatability and
Description.
Huaiyang Huang, Haoyang Ye, Yuxiang Sun, Ming Liu.
IEEE International Conference on Robotics and Automation (ICRA)
, 2020.